Keynote Speakers
Keynote Speakers
TBA
Professor Angelo Cangelosi
University of Manchester and Alan Turing Institute, UK
Title: Developmental Robotics for Language Learning, Trust and Theory of Mind
Abstract: Growing theoretical and experimental research on action and language processing and on number learning and gestures clearly demonstrates the role of embodiment in cognition and language processing. In psychology and neuroscience, this evidence constitutes the basis of embodied cognition, also known as grounded cognition (Pezzulo et al. 2012). In robotics and AI, these studies have important implications for the design of linguistic capabilities in cognitive agents and robots for human-robot collaboration, and have led to the new interdisciplinary approach of Developmental Robotics, as part of the wider Cognitive Robotics field (Cangelosi & Schlesinger 2015; Cangelosi & Asada 2022). During the talk we will present examples of developmental robotics models and experimental results from iCub experiments on the embodiment biases in early word acquisition and grammar learning (Morse et al. 2015; Morse & Cangelosi 2017) and experiments on pointing gestures and finger counting for number learning (De La Cruz et al. 2014). We will then present a novel developmental robotics model, and experiments, on Theory of Mind and its use for autonomous trust behavior in robots (Vinanzi et al. 2019, 2021). The implications for the use of such embodied approaches for embodied cognition in AI and cognitive sciences, and for robot companion applications will also be discussed.
Short Bio: Angelo Cangelosi is Professor of Machine Learning and Robotics at the University of Manchester (UK) and co-director and founder of the Manchester Centre for Robotics and AI. He was selexcted for the award of the European Research Council (ERC) Advanced grant (funded by UKRI). His research interests are in cognitive and developmental robotics, neural networks, language grounding, human robot-interaction and trust, and robot companions for health and social care. Overall, he has secured over £38m of research grants as coordinator/PI, including the ERC Advanced eTALK, the UKRI TAS Trust Node and CRADLE Prosperity, the US AFRL project THRIVE++, and numerous Horizon and MSCAs grants. Cangelosi has produced more than 300 scientific publications. He is Editor-in-Chief of the journals Interaction Studies and IET Cognitive Computation and Systems, and in 2015 was Editor-in-Chief of IEEE Transactions on Autonomous Development. He has chaired numerous international conferences, including ICANN2022 Bristol, and ICDL2021 Beijing. His book “Developmental Robotics: From Babies to Robots” (MIT Press) was published in January 2015, and translated in Chinese and Japanese. His latest book “Cognitive Robotics” (MIT Press), coedited with Minoru Asada, was recently published in 2022.
Professor Haris Mouratidis
Director of Institute for Analytics and Data Science – IADS, Professor of Data Science and Statistics, School of Computer Science and Electronic Engineering, University of Essex, UK
Title: AI and cybersecurity: Friend or Foe?
Abstract: We live in an era of unprecedented technological advancement that has an impact on every aspect of the human life. Within that environment, artificial intelligence and cybersecurity are two areas where innovation and challenges intersect with profound implications. On one hand, AI, with its transformative capabilities, is revolutionising how we process information, make decision and interact with technology, while on the other hand, cybersecurity provides essential tools to safeguard the digital infrastructures that we depend on.
In this talk I will discuss the interplay between the two, exploring both the benefits of using AI for cybersecurity and cybersecurity for AI, but also the challenges that such co-existence introduces. Drawing on real-world case studies and insights, I will discuss how machine learning, threat detection and analytics can empower organisations and individuals to improve their cybersecurity but also how AI-driven tactics give rise to sophisticated cyber threats. I will then emphasize the necessity for collaborative initiatives spanning both AI and cybersecurity domains. I will stress the importance of continuous shared research and education to foster an environment where the coexistence of AI and cybersecurity not only enhances our digital landscape but also minimizes associated risks
Short Bio: Haralambos (Haris) Mouratidis is Professor and Director of the Institute for Analytics and Data Science (IADS) at the University of Essex. Before, he was professor of secure software engineering and founding director of the Centre for Secure, Intelligent and Usable System (CSIUS) at the University of Brighton. His research interests include cybersecurity data science (with focus on AI and machine learning and data analytics for cybersecurity risk management, threat modelling and data protection), intelligent data security engineering (with focus on the development of novel methodologies and techniques to improve privacy by design and security by design and AI-enabled model based security engineering), and Threat modelling and privacy protection for AI and data science (with focus on adversarial attacks on machine learning and machine learning facilitated adversarial mechanisms). He has published more than 210 papers and he has secured funding of c.£30M, funded mostly by the UK and the EU. He is Fellow of the UK Higher Education Academy and “Standards-maker” of the British Standards Institution for the “Privacy-By-Design” and “Software and Systems Engineering” national committees. He is elected Vice-Chair of the IFIP WG on Secure Engineering, Expert Fellow of the UK Digital Economy Network Plus, on the register of ENISA’s experts and was member of the ENISA WG on European Cybersecurity Skills Framework (ECSF). He has been invited subject expert for events organised by national and international organisations (e.g. EU, NATO); more recently he spoke about AI-driven Privacy-by-Design, on an event organised by the European Commission and led discussion on challenges of Automated Software Engineering towards GDPR compliance at a meeting organised by the European Research Executive Agency.
Professor Emma Hart
FRSE, Professor at Edinburgh Napier University, Edinburgh, Scotland, United Kingdom
Title: An Evolutionary Approach to the Autonomous Design and Fabrication of Robots for Operation in Unknown Environments
Abstract: Robot design is traditionally the domain of humans – engineers, physicists, and increasingly AI experts. However, if the robot in intended to operate in a completely unknown environment (for example clean up inside a nuclear reactor) then it is very difficult for human designers to predict what kind of robot might be required. Evolutionary computing is a well-known technology that has been applied in various aspects of robotics for many years, for example to design controllers or body-plans. When coupled with advances in materials and printing technologies that allow rapid prototyping in hardware, it offers a potential solution to the issue raised above, for example enabling colonies of robots to evolve and adapt over long periods of time while situated in the environment they have to work in. However, it also brings new challenges, from both from an algorithmic and engineering perspective.
The additional constraints introduced by the need for example to manufacture robots autonomously, to explore rich morphological search-spaces and develop novel forms of control require some re-thinking of “standard’ approaches in evolutionary computing, particularly on the interaction between evolution and individual learning. I will discuss some of these challenges and propose and showcase some methods to address them that have been developed in during a recent project
Short Bio: Professor Emma Hart has worked in the field of Evolutionary Computing for over 20 years. Her current work is mainly centred in Evolutionary Robotics, bringing together ideas on using artificial evolution as tool for optimisation with research that focuses on how robots can be autonomously designed and fabricated, and endowed with the ability to continually learn over a lifetime, improving performance as they gather information from their own or other robots’ experiences. The work has attracted significant media attention including recently in the New Scientist, and the Guardian. She gave a TED talk on this subject at TEDWomen in December 2021 in Palm Springs, USA which has attracted over 1 million views since being released online in April 2022. She was the Editor-in-Chief of the journal Evolutionary Computation (MIT Press) from 2017-2024 and an elected member of the ACM SIG on Evolutionary Computing. In 2022, she was honoured to be elected as a Fellow of the Royal Society of Edinburgh for her contributions to the field of Computational Intelligence and was awarded the ACM SIGEVO Award for Outstanding Contribution to Evolutionary Computation in 2023.
Professor Plamen Angelov
Lancaster University, UK
Title: Interpretable-by-design prototype-based deep learning
Abstract: Deep Learning justifiably attracted the attention and interest of the scientific community and industry as well as of the wider society and even policy makers. However, the predominant architectures (from Convolutional Neural Networks to Transformers) are hyper-parametric models with weights/parameters being detached from the physical meaning of the object of modelling. They are, essentially, embedded functions of functions which do provide the power of deep learning; however, they are also the main reason of diminished transparency and difficulties in explaining and interpreting the decisions made by deep neural network classifiers. Some dub this “black box” approach. This makes problematic the use of such algorithms in high stake complex problems such as aviation, health, bailing from jail, etc. where the clear rationale for a particular decision is very important and the errors are very costly. This motivated researchers and regulators to focus efforts on the quest for “explainable” yet highly efficient models. Most of the solutions proposed in this direction so far are, however, post-hoc and only partially addressing the problem. At the same time, it is remarkable that humans learn in a principally different manner (by examples, using similarities) and not by fitting (hyper-) parametric models, and can easily perform the so-called “zero-shot learning”. Current deep learning is focused primarily on accuracy and overlooks explainability, the semantic meaning of the internal model representation, reasoning and decision making, and its link with the specific problem domain. Once trained, such models are inflexible to new knowledge. They cannot dynamically evolve their internal structure to start recognising new classes. They are good only for what they were originally trained for. The empirical results achieved by these types of methods according to Terry Sejnowski “should not be possible according to sample complexity in statistics and nonconvex optimization theory”. The challenge is to bring together the high levels of accuracy with the semantically meaningful and theoretically sound and provable solutions.
All these challenges and identified gaps require a dramatic paradigm shift and a radical new approach. In this talk, the speaker will present such a new approach towards the next generation of explainable-by-design deep learning. It is based on prototypes and uses kernel-like functions making it interpretable-by-design. It is dramatically easier to train and adapt without the need for complete re-training, can start learning from few training data samples, explore the data space, detect and learn from unseen data patterns. Indeed, the ability to detect the unseen and unexpected and start learning this new class/es in real time with no or very little supervision is critically important and is something that no currently existing classifier can offer. This method was applied to a range of applications including but not limited to remote sensing, autonomous driving, health and others.
Short Bio: Professor Plamen Angelov holds a Chair in Intelligent Systems and is Director of Research at the School of Computing and Communications. He is founding Director of the Lancaster Intelligent, Robotic and Autonomous systems (LIRA) Centre (www.lancaster.ac.uk/lira ) which brings together 70+ academics/faculty across 15 Departments of the University. Prof. Angelov is a Fellow of the IEEE, of the IET and of ELLIS (https://ellis.eu) and a Governor of the International Neural Networks Society (INNS) for which he also served two consecutive terms as Vice President (2017-2020). He has over 350 publications in leading journals (like TPAMI, Information Fusion, IEEE Transactions on Cybernetics, on Fuzzy Systems, etc.), peer-reviewed conference proceedings (such as CVPR, IEEE), 3 granted US patents, 3 research monographs (by Wiley, 2012 and Springer, 2002 and 2018) cited over 12800 times with an h-index of 60. He has an active research portfolio in the area of interpretable (explainable-by-design) deep learning and internationally recognised results into explainable deep learning, evolving systems for streaming data and computational intelligence. Prof. Angelov leads numerous projects funded by UK research councils, EC, European Space Agency, DSTL, GCHQ, Royal Society, Faraday Institute, industry. He is recipient of the Dennis Gabor award (2020) for “outstanding contributions to engineering applications of neural networks”, IEEE awards ‘For outstanding Services’ (2013 and 2017) and other awards. He is Editor-in-Chief of Springer’s journal Evolving Systems and Associate Editor of IEEE Transactions on Cybernetics, IEEE Transactions on Fuzzy Systems, IEEE Transactions on AI and other journals. He gave 30+ keynote talks and was General co-Chair of a number of high profile IEEE conferences. He is founding Chair of the Technical Committee on Evolving Intelligent Systems, SMC Society of the IEEE and was previously chairing the Standards Committee of the Computational Intelligent Society of the IEEE (2010-2012) where he initiated and chaired the Work Group P2976 on the IEEE standard on explainable AI. He is the founding co-Director of one of the funded programmes by ELLIS (on Human-centred machine learning). He was also a member of International Program Committee of over 150 international conferences (primarily IEEE).
Professor Oresti Baños Legrán
Tenured Professor of Computational Behaviour Modelling, Department of Computer Engineering, Automation and Robotics, Research Centre for Information and Communications Technology, University of Granada, Spain
Title: Intelligent mobile sensing for understanding human behaviour.
Abstract: Understanding people’s behaviour is essential to characterise patient progress, make treatment decisions and elicit effective and relevant coaching actions. Hence, a great deal of research has been devoted in recent years to the automatic sensing and intelligent analysis of human behaviour. Among all sensing options, smartphones stand out as they enable the unobtrusive observation and detection of a wide variety of behaviours as we go about our physical and virtual interactions with the world. This talk aims at giving the audience a taste of the unparalleled potential that mobile sensing in combination with artificial intelligence offers for the study of human individual and collective behaviour.
Short Bio: Oresti Baños is an Associate Professor of Computational Behaviour Modelling at the University of Granada (Spain, 2019-present). He is also a Senior Research Scientist affiliated with the Centre for Information and Communication Technologies of the University of Granada (CITICUGR). He is a Research Collaborator at the University of Twente (Netherlands, 2018-present). He is a former Assistant Professor of Creative Technology and a Telemedicine Research Scientist at University of Twente (Netherlands, 2016-2018). Here, he worked for the Biomedical Signal and Systems Group (BSS), the Centre for Telematics and Information Technology (CTIT), the Research Centre for Biomedical Technology and Technical Medicine (MIRA), and the Centre for Monitoring and Coaching (CMC). He was a Postdoctoral Research Fellow at Kyung Hee University (South Korea, 2014-2016), a Predoctoral Research Fellow at the CITIC-UGR (Spain, 2010-2014) and a Visiting Scholar at the Technical University of Eindhoven (Netherlands, 2012), the Swiss Federal Institute of Technology Zurich (Switzerland, 2011), and the University of Alabama (USA, 2011). He holds three Master’s degrees in telecommunications (2009), electrical (2011) and computer networking engineering (2011) and a PhD in computer science (2014), all with honors, from the University of Granada. Most recently, he has been Principal Investigator of the Dutch R&D project HoliBehave, Workpackage Lead of the EU-H2020 project COUCH and Technical Coordinator of the Korean CV date 24/09/2019 2 project Mining Minds. He has participated in more than 20 Spanish, Korean, Dutch and FP7/H2020 European digital health and behavioural computing-related projects such as HPCBIO, AdaBIO, DSIPA-BIO, AmIVital, Symbionics, OPPORTUNITY, XOSOFT, eNHANCE or COUCH. He is author of more than 80 papers, most of them indexed in top-ranked international conferences and journals. He is co-organiser of several digital health workshops such as SUT4Coaching or Health-i-Coach and he is also a member of the organising committee of a number of international conferences including PervasiveHealth, IWBBIO or UCAmI.
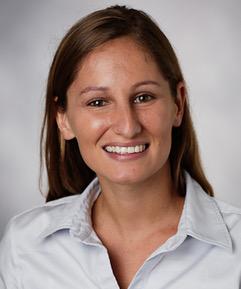
Professor Tamara Silbergleit Lehman
Assistant Professor at University of Colorado Boulder
Title: Secure, Efficient and High Performance Computing: A Computer Architecture Perspective
Abstract: Distributed systems and new architectures introduce new sets of security risks. Microarchitectural attacks have presented many challenges in the computer architecture community and this talk will present a few of the methods that the Boulder Computer Architecture Lab (BCAL) has been studying in order to address these vulnerabilities. The talk will first introduce physical and microarchitectural attacks and why they are hard to mitigate. Then, the talk will introduce an efficient implementation of speculative integrity verification, Poisonivy, to construct an efficient and high performance secure memory system. Finally, the talk will show how we can leverage emerging memory technologies such as near memory processing to defend and identify microarchitectural side-channel attacks. The talk will end by briefly introducing a new research direction that is investigating the Rowhammer attack impact on neural network accuracy running on GPUs and how we can leverage secure memory to protect the accuracy of the models.
Short Bio: Tamara Silbergleit Lehman is an assistant professor at the University of Colorado Boulder in the Electrical, Computer and Energy Engineering Department. She also holds a courtesy appointment in the Computer Science department. She is a member of the Colorado Research Center for Democracy and Technology. Her work focuses on all aspects of computer security from the hardware perspective. Her research interests span a wide array of topics on the intersection of computer architecture and security. Before doing her PhD, she completed a Masters of Engineering degree at Duke University in the Electrical and Computer Engineering Department and a Bachelor of Science from the University of Florida in Industrial and Systems Engineering. She is passionate about computer architecture, and her industrial engineering background gives her a new perspective on ways to optimize systems. She also enjoys working on the security space because it is one of the most challenging problems facing the computer industry. At the heart of most computer security challenges is people. Computers do what they are designed to do, but it is the people who re-define the system’s functionality. She strongly believes that secure systems should not rely on people writing correct code, or running well-intended applications, but instead having well defined functionality with well defined side-effects. In addition, her research is guided by the principle that all computers should be secure and efficient. Systems should not have to sacrifice efficiency for security.
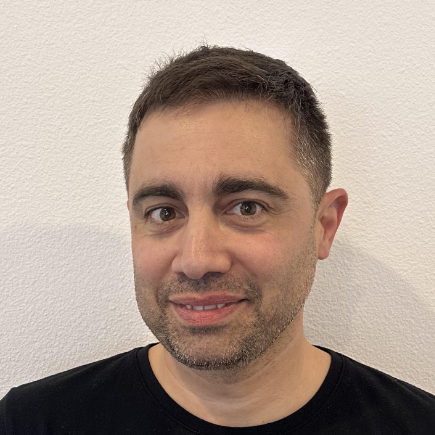
Dr. Javier Alonso Lopez
Principal Machine Learning Applied Scientist Microsoft AI Platform – Microsoft OpenAI
Title: How AI/Machine Learning has the power of revolutionizing (for good?) cybersecurity?
Abstract: As we already know, Machine Learning is already used in various cybersecurity tasks such as malware identification/classification, intrusion detection, botnet identification, phishing, predicting cyberattacks like denial of service, fraud detection, etc. However, during the last years there has been a revolution of machine learning, specifically, deep learning that creates not only an unbelievable opportunity to develop more effective solutions but also represents a new threat and a new tool to be used to attack and gain control over systems, organizations and even countries.
In this talk, we will overview the major applications of Machine Learning in the field of cybersecurity both to prevent attacks but also how Machine learning can be used to pose a threat. We will review the main advances of Deep Learning in the last 5 years and their application into Cybersecurity. Finally, we will discuss the possible future trends we can expect (I do not expect a high accuracy, but high recall :D) in the intersection of Deep Learning and Cybersecurity.
Short Bio: Dr. Javier Alonso received his master’s degree in Computer Science degree and his Ph.D. degree from the Technical University of Catalonia (Universitat Politecnica de Catalunya, UPC) in 2004 and 2011, respectively. From 2006 to 2011, he held an assistant lecturer position in the Computer Architecture Department of UPC. From 2011 to 2015 he held a Postdoctoral Associate position and later Research Assistant Professor at the RIASC Lab led by Professor K.S. Trivedi, in the Electrical and Computer Engineering Department, Duke University, Durham, NC, USA. From 2015 to 2016 he was leading the research at the just created Research Institute of Applied Sciences in Cybersecurity as acting research director at the University of Leon, Spain. After academia, Dr. Alonso decided to move to industry and joined Amazon as a Senior Machine Learning scientist working in a myriad of verticals applying Machine and Deep Learning in diverse fields like e-commerce, drone delivery program (8+ US Patents) and transportation. After almost 7 years at Amazon, in 2023 Dr. Alonso joined Microsoft AI platform organization as Principal Machine Learning Applied Scientist to work on Microsoft OpenAI initiative helping Microsoft customers to adopt OpenAI Large Language Models (LLMs).
During his career, Dr. Alonso has published 40+ papers about different aspects of applied Machine learning to areas like dependability, performance, cybersecurity, geospatial and path planning just naming a few. He has filed 10+ US Patents (6 already granted) in the intersection between Deep Learning, geospatial and Drones. He has also contributed to the adoption of Machine Learning across different roles at Amazon as being one of the first instructors at the Amazon Machine Learning University. He has also served as a reviewer for IEEE Transactions on Computers, IEEE Transactions on Dependability and Security Computing, Performance Evaluation, and Cluster Computing, and several IEEE and ACM international conferences. During his career Dr. Alonso has been involved in multiple research and applied projects founded by organizations like NASA, JPL/NASA, NEC Japan, NATO, Huawei, or WiPro to name a few. His research interests are focused on the application of Machine Learning/Deep Learning across different fields.